Join WTS-NH for a virtual Lunch n' Learn on the topic of Improving Reliability of Roadway Asset Data Collected by Vehicle-Based Platforms.
April 19th, 2023
Noon- 1:00 pm ET
Virtual event with PDH credit hours available
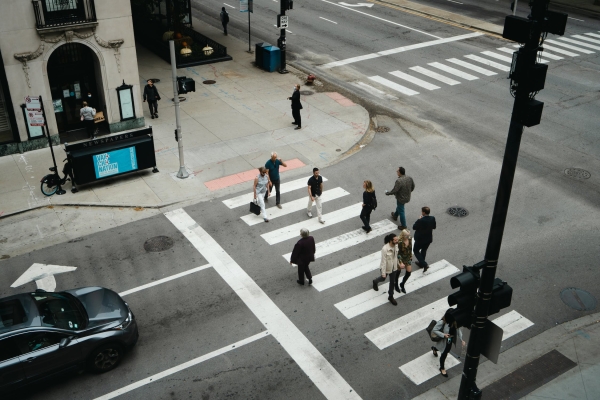
More information about the upcoming Lunch n' Learn: Roadside asset data such as line striping, traffic signs, pavement messages, sidewalks, medians, manholes, etc. is of high importance for public transportation agencies to maintain a healthy and efficient transportation network. In recent years, collection, inventorying and assessing these assets have become of growing importance to improve the overall transportation infrastructure alongside pavements. Recording and monitoring assets has been traditionally conducted through manual field inspections which requires considerable time and cost. In past decade, there has been increasing interest in automated asset data collection methods using LiDAR (Light Detection and Ranging) and extraction of the assets using machine learning and artificial intelligence techniques. Given the volume of data generated and inherent quality issues from automated extraction methods, an efficient and comprehensive quality control process is required to generate reliable data.
This paper presents a statistical-based approach for conducting comprehensive quality control on transportation asset data and improving reliability of the extracted data. In this approach, libraries of data patterns were created and programmed for each specific asset. The library of patterns continues to develop as more data is available. The extracted raw data is analyzed and compared to these rules and patterns. The sections that do not fit the guidelines of the set patterns are flagged for further review. This approach is a systematic approach to review the entire dataset and flag candidates for manual review rather than selecting random sections for quality control purposes. This study in based on an implementation which includes 25 roadway asset classes for approximately 3000 lane miles of roadway.
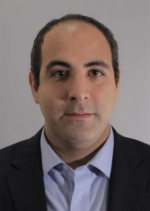
Meet our presenter: Amir Arshadi, (AECOM) is a civil engineer with over ten years of experience in the transportation infrastructure industry and research. His background involves several projects on transportation data analysis, pavement design, construction, and management, big data mining, and software development. He serves various federal, state, and commercial clients across the country by applying advanced technological solutions in monitoring, evaluation, and analysis. Amir is a strong technical communicator specializing in data science, transportation asset management, pavement engineering, and project delivery.
Thank you to our sponsor, AECOM, and our presenter Amir Arshadi.
Interested in joining this Lunch n' Learn event? Contact Delia Makhetha at [email protected] for the virtual login information.